The OpenSMOKE++ framework was used in the context of adaptive reduced chemistry for multidimensional laminar flames in a recent publication on Energies with title: “Impact of the partitioning method on multidimensional adaptive-chemistry simulations”. The work is a collaboration between the CRECK Modeling Lab and the BURN Group at Universite’ Libre de Bruxelles.
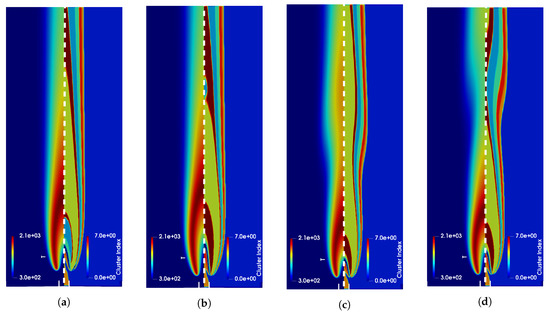
G. D’Alessio, A. Cuoci, G. Aversano, M. Bracconi, A. Stagni, A. Parente, Impact of the partitioning method on multidimensional adaptive-chemistry simulations, (2020), Energies, 13(10), no. 2567, DOI: 10.3390/en13102567
Abstract
The large number of species included in the detailed kinetic mechanisms represents a serious challenge for numerical simulations of reactive flows, as it can lead to large CPU times, even for relatively simple systems. One possible solution to mitigate the computational cost of detailed numerical simulations, without sacrificing their accuracy, is to adopt a Sample-Partitioning Adaptive Reduced Chemistry (SPARC) approach. The first step of the aforementioned approach is the thermochemical space partitioning for the generation of locally reduced mechanisms, but this task is often challenging because of the high-dimensionality, as well as the high non-linearity associated to reacting systems. Moreover, the importance of this step in the overall approach is not negligible, as it has effects on the mechanisms’ level of chemical reduction and, consequently, on the accuracy and the computational speed-up of the adaptive simulation. In thiswork, two different clustering algorithms for the partitioning of the thermochemical space were evaluated by means of an adaptive CFD simulation of a 2D unsteady laminar flame of a nitrogen-diluted methane stream in air. The first one is a hybrid approach based on the coupling between the Self-Organizing Maps with K-Means (SKM), and the second one is the Local Principal Component Analysis (LPCA). Comparable results in terms of mechanism reduction (i.e., the mean number of species in the reduced mechanisms) and simulation accuracy were obtained for both the tested methods, but LPCA showed superior performances in terms of reduced mechanisms uniformity and speed-up of the adaptive simulation. Moreover, the local algorithm showed a lower sensitivity to the training dataset size in terms of the required CPU-time for convergence, thus also being optimal, with respect to SKM, for massive dataset clustering tasks.